Machine Learning Optimizes Aluminum Heat Exchanger Production
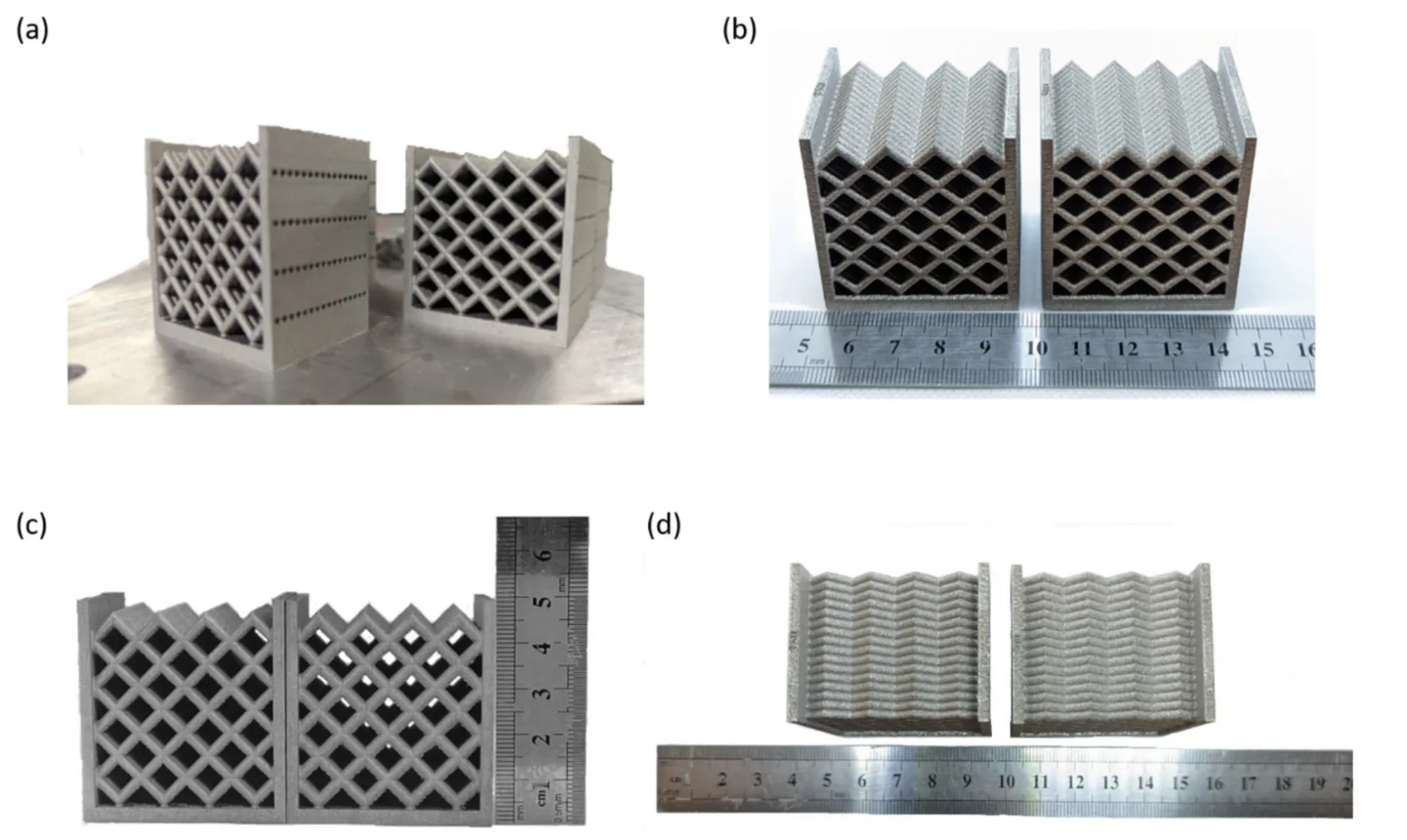
A recent research paper highlights how machine learning can optimize the additive manufacturing of an advanced aluminum alloy heat exchanger. Authored by NSIRC and University of Birmingham PhD student Francesco Careri, the study describes a Powder Bed Fusion-Laser Beam on Metal (PBF-LB/M) process guided by machine learning. The approach tackled metallurgical defects, dimensional accuracy, and surface quality by using neural networks to identify ideal parameters.
Initial tests generated a process map for the A205 aluminum alloy, investigating how variations in laser parameters, beam compensation, and contour distance affect manufacturing outcomes. The optimization phase reduced thickness deviations in thin-walled lattice structures to under 2%, enabling successful fabrication of a prototype heat exchanger. This achievement suggests a feasible path toward producing complex heat exchangers with minimal porosity.
The study, co-authored with Dr. Leonardo Stella and Professor Moataz Attallah of the University of Birmingham, illustrates how integrating machine learning with PBF-LB/M supports the creation of intricate, defect-free parts. TWI’s mentorship and collaboration also underscore ongoing efforts to develop new solutions and train the next generation of specialists in advanced manufacturing technologies.
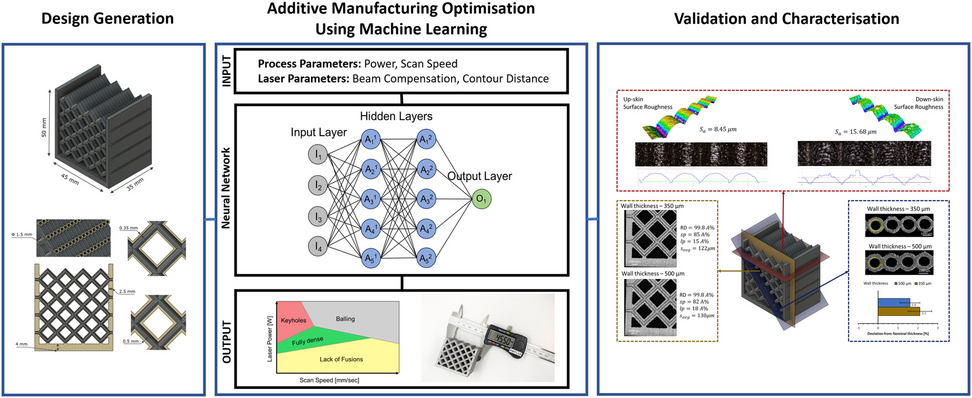
Discussion