HAMS-Net: A New Approach to Weld Seam Classification
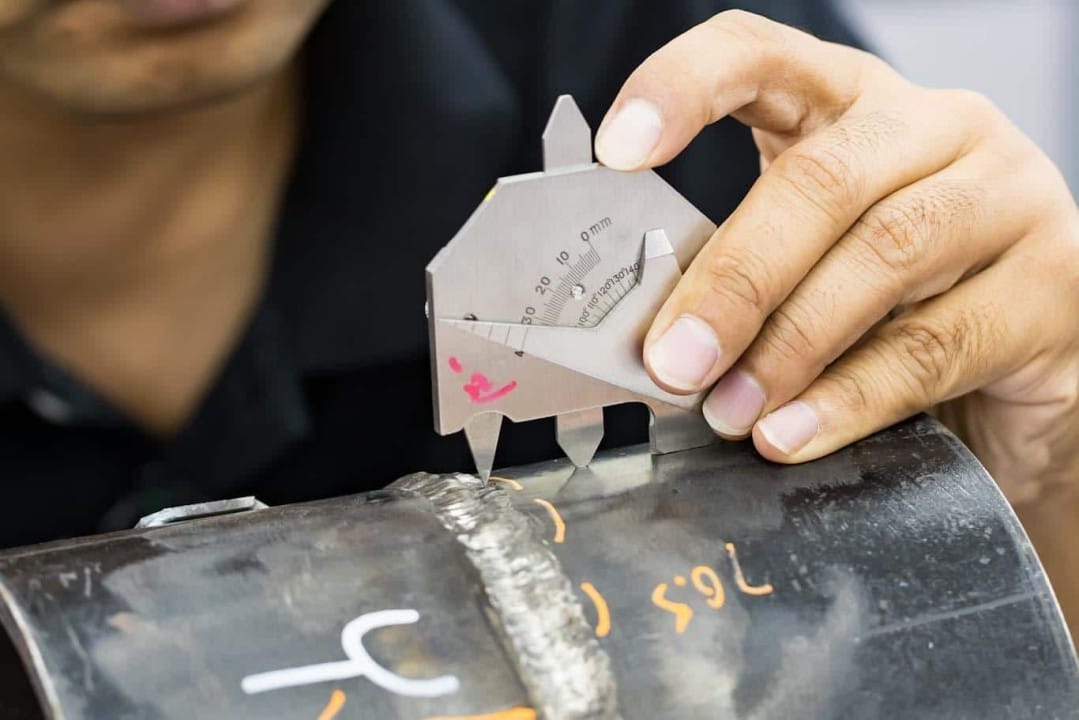
HAMS-Net, short for Heterogeneous Attention Multi-Scale Network, is drawing attention in the welding community for its approach to classifying weld seams. Developed as a way to handle complex weld geometries and subtle differences between weld types, this framework incorporates innovative modules like Channel-Spatial Attention and Heterogeneous Attention Pooling. According to the researchers, these features help the network focus on critical weld characteristics and capture multi-scale information efficiently.
In testing across multiple datasets, including specialized industrial sets, HAMS-Net demonstrated improved accuracy and faster processing when compared to models like Swin-Transformer and ViT. The authors emphasize that it not only excels in distinguishing subtle weld defects but also remains robust under challenging conditions such as noise and data loss. Additionally, the adaptive design is intended to make HAMS-Net suitable for real-time industrial settings, addressing both performance and computational constraints.
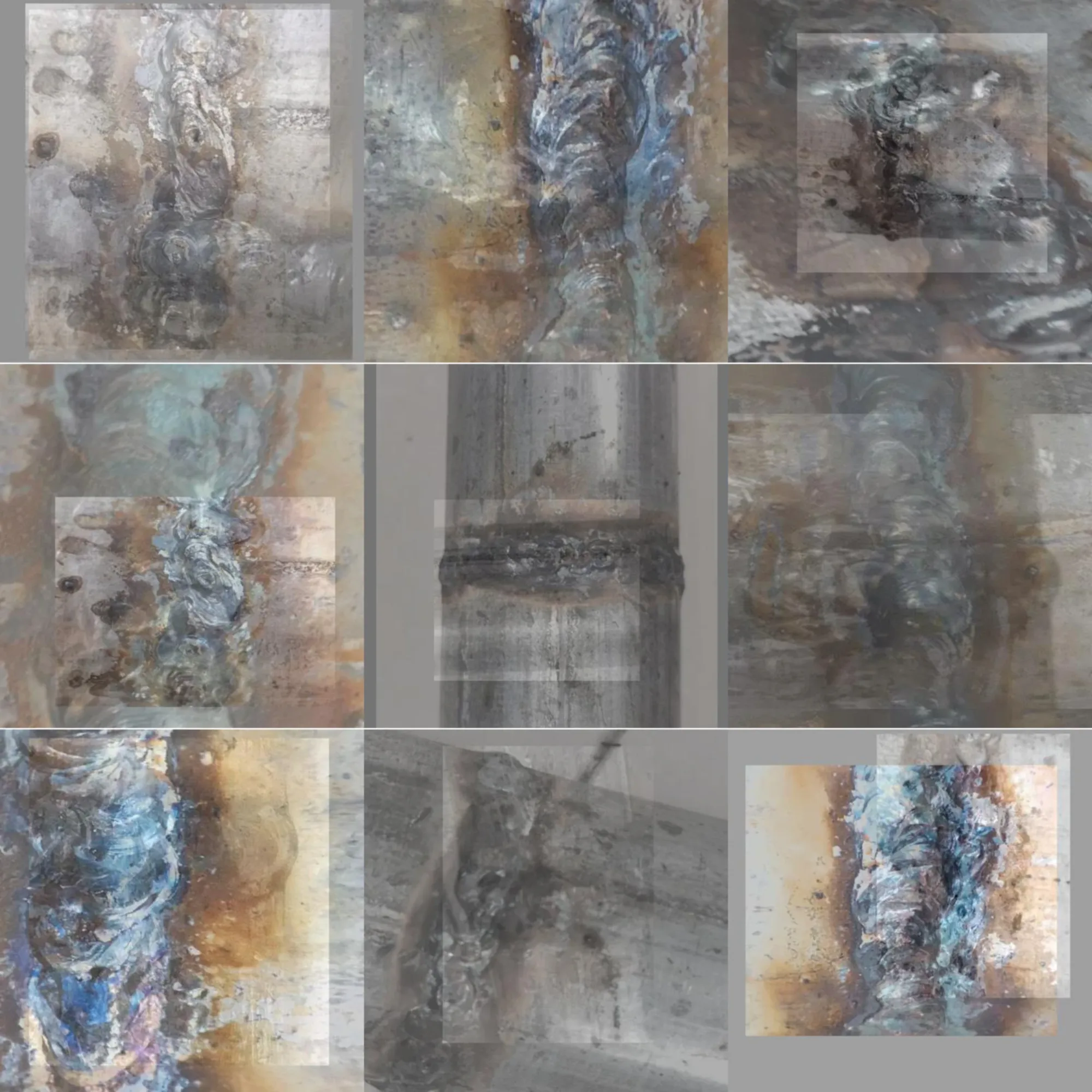
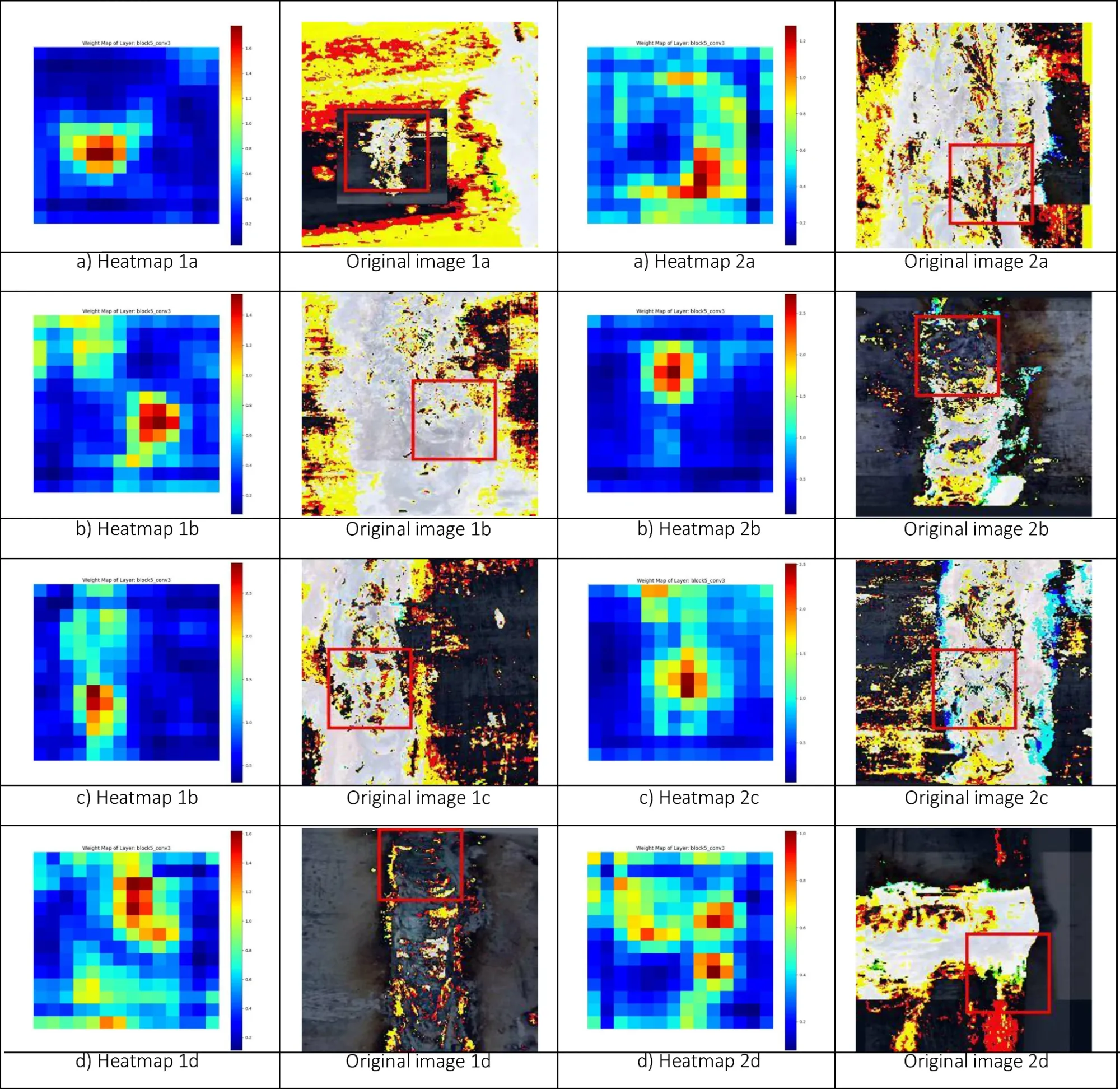
Fig.1 - Dataset image example, Fig.2 - Weld feature visualization.
The research team points to HAMS-Net’s potential to streamline automated weld inspections by reducing errors and processing overhead. Beyond its accuracy scores, the model aims to accommodate the wide variety of weld types seen in modern production lines. While the full code and dataset details can be obtained from the corresponding author, the published findings indicate that this method may help young welders and experienced professionals alike by providing a more reliable tool for weld seam classification.
Source:
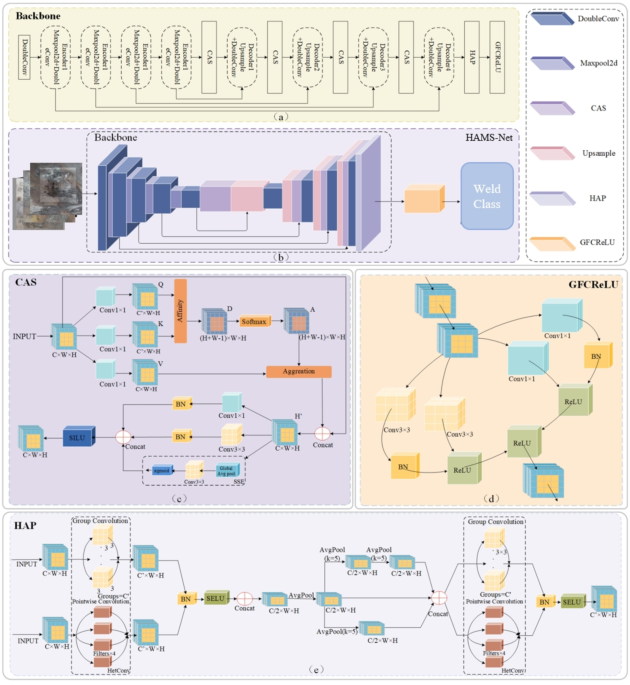
Discussion